Speakers
Energy & Environment
Fotis Anagnostopoulos
Fotis Anagnostopoulos was born in 1993 in Athens, from Arcadia. He received a B.Sc. degree in Physics from the University of Ioannina, an M.Sc. degree in the program “Astrophysics, Astronomy, Engineering” of the National Kapodistrian University of Athens, where he completed his PhD. The latter concerns the study of the accelerated expansion of the Universe. His research interests include modified theories of gravity, Machine Learning and Fluid Dynamics. In total, he has published 15 research papers in reputed journals such as Physical Review D, Journal of Cosmology & Astroparticle Physics etc., which have received 426 citations. The subject of his postdoctoral work at the University of Peloponnese is the study of microparticles in urban environments, with emphasis on port cities. He is currently working on the implementation of a general method for the prediction of microparticle concentration, with the ultimate goal of implementing the method as a service freely available to the general public.
Air pollution prediction models using machine learning methods
Air pollution is a serious risk to human health in the urban environment, so the ability to predict pollutant concentrations is necessary, along with pollution control actions. In this direction, transport models are used internationally, but they have accuracy limitations when applied to the urban environment. We present a new approach to the problem using Artificial Neural Networks (ANNs), namely Long Short-Term Memory Neural Networks (LTSMNs), which are known for their high accuracy in time series prediction problems. The specific MBMNs used have the additional innovative feature of being able to interpret their results. Regarding the used forecasting characteristics, meteorological data, past concentration values and, in addition, urban characteristics such as the Average Building Factor and population density are exploited. The use of the latter allows for a better capture of the spatial variability of the phenomenon, while providing the possibility to add sensors or to integrate moving sensors on drones or buses, which was not easy based on the approaches used so far. The method is applied in a number of Greek cities (Patras, Agrinio, Athens, etc.) and is found to provide prediction accuracy comparable to the sensor’s discriminatory capability, while maintaining the possibility of interpreting the contribution of individual features to the prediction. Therefore, the proposed method can form the core of an integrated low-cost early warning system that can contribute to the reduction of the health risk caused by air pollution.
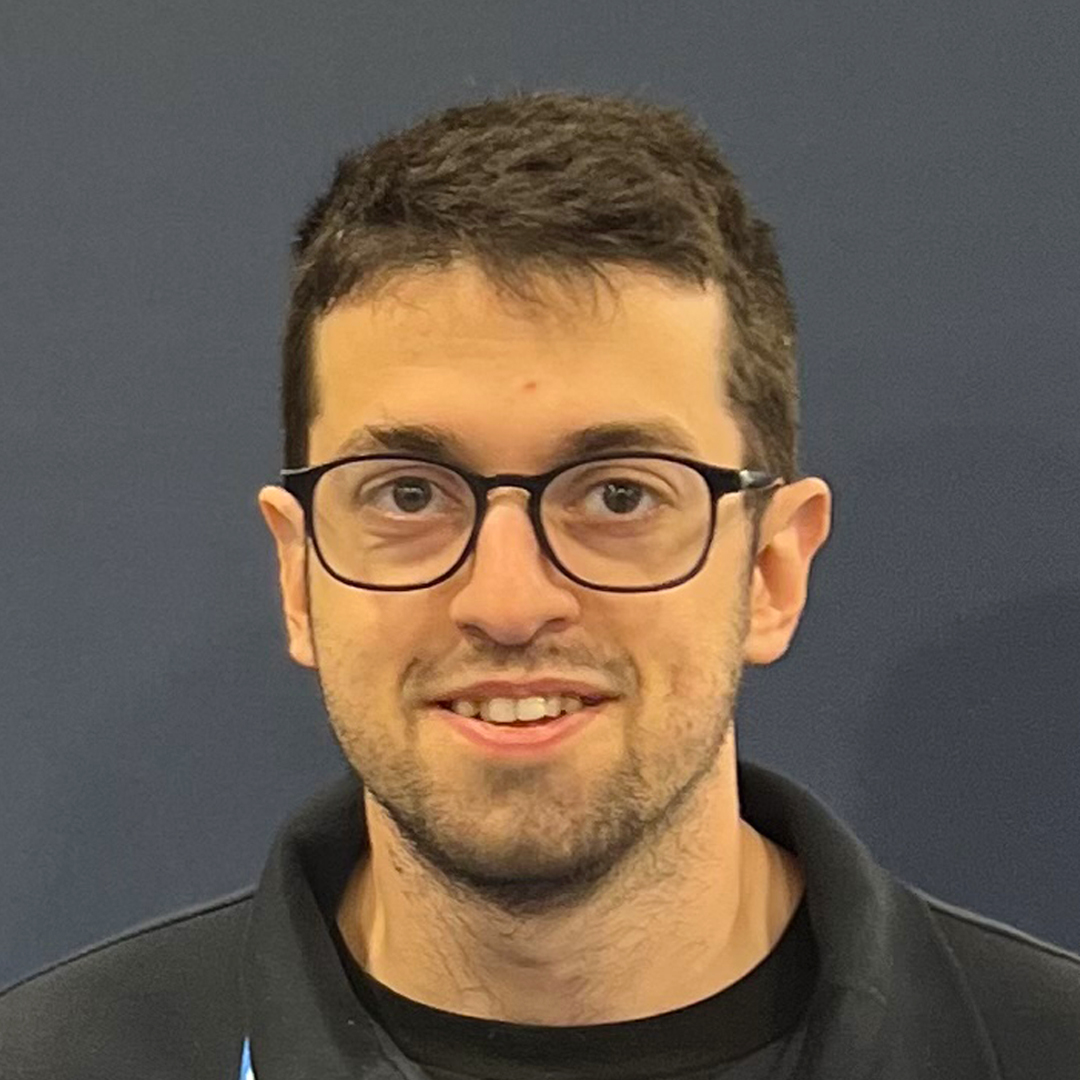